What is data minimisation?
Data minimisation is defined in the GDPR legislation as follows:
The principle of “data minimisation” means that a data controller should limit the collection of personal information to what is directly relevant and necessary to accomplish a specified purpose. They should also retain the data only for as long as is necessary to fulfil that purpose. In other words, data controllers should collect only the personal data they really need, and should keep it only for as long as they need it.
The data minimisation principle is expressed in Article 5(1)(c) of the GDPR and Article 4(1)(c) of Regulation (EU) 2018/1725, which provide that personal data must be "adequate, relevant and limited to what is necessary in relation to the purposes for which they are processed".- source
In short – Only capture the personal data you need and store it as long as you need it.
Reasons for minimising data
Even though it might be tempting to capture as much as possible within the boundaries of the law and given you have consent to do so, the principle of data minimisation should be applied continuously in order to consistently minimise the amount of data captured. So, why should data be minimised rather than following a capture-all approach?
Protect your visitors, prospects and customersOver the last years data theft has become a new threat and reality for businesses. Carissa Véliz reminds us of the following in her book “privacy is power” - “Institutions that hoard more data than is necessary are generating their own risk”.Knowing that the risk of an attempt of data theft is real it is of uttermost importance to protect the anonymity of the data subjects as much as possible. After all, it is the data controller who is responsible for the data at all times.
Minimising your data as much as possible with data minimisation techniques reduces the value of the data if a breach or leak would occur.
Reduce your carbon footprint The World Economic Forum estimates that single use data is responsible for 2.5% of all human-induced carbon dioxide. Data minimisation can help in a number of ways to reduce this number. First of all by ensuring as little as possible single use data is being captured. A large part of the single use data can be considered as “nice to have data” but with limited business value. Secondly the removal of data after its useful lifetime has elapsed would reduce data centre carbon dioxide emissions drastically.
Protect your wallet. Data that is not used still accounts for a cost. As the speed and volume at which data is being captured increased, so is the cost. It is therefore wise to evaluate if this single use data is worth the long term cost.
The next question is – What is the pragmatic approach to apply data minimisation for analysts?
Data minimisation principles applied to your tracking plans
When it comes to building tracking plans two ideas that facilitate data minimisation need to be kept in mind:
It’s not because you can that you should
Aggregate whenever possible
It’s not because you can that you should
Imagine the following user story:"As a product manager I would like to have a proper overview on how effective CTA's are at driving sign-ups.”
Now also take into account that the user has given consent for his/her data to be captured for analytics purposes.
The customer journey looks as follows and as a result we could assume that each of these white boxes represents an event and each of the yellow post-it represents event properties.
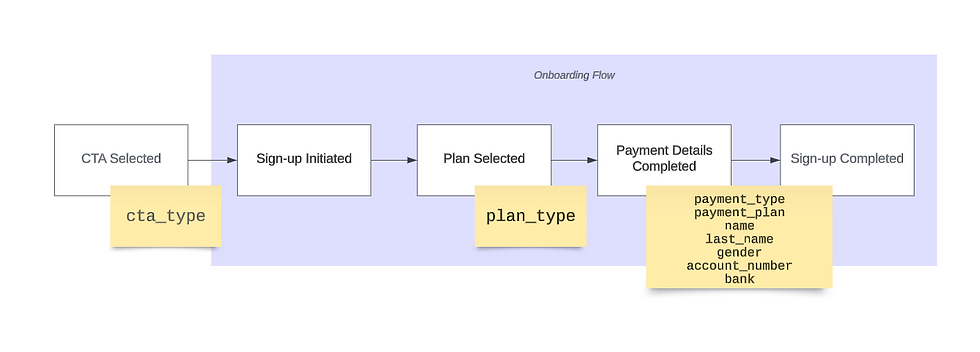
But do we need all of this data? Theoretically we could capture it as we have consent from the user. However, our use case does not require it.
Alternatively the tracking plan could look as follows where the red fields have been omitted from the tracking plan.

The use case can be solved using the events and properties that are left. Our total amount of data captures has been reduced, and more importantly, redundant fields that are sensitive (PII) have been omitted entirely. As a result our risk, carbon footprint and monetary cost have been reduced.
Aggregate whenever possible
Personalisation use cases usually require precision metrics when it comes to PII, analytics use cases usually work using aggregated metrics.
Imagine the following use case:
"As a product manager I would like to understand what the age is of our customers who purchase the premium version of our product.”
This would require us to capture a certain amount of PII - age.
As a result most analysts would like to capture and store the precise age of the customer in order to be able to conduct this analysis. However, does it matter much in terms of actionability if we know that the average age is between [31-35] versus if we know that it is 32? Probably not.
However, it does offer more protection for a customer whose records are leaked when the age indicates [31-35] rather than precisely 30 years old and 27 days. As a result data aggregation can make re-identification harder when records get leaked while preserving the usefulness of the data to fulfil the use case. So whenever PII needs to be part of your tracking plans in order to support specific use cases, aggregate the data whenever possible.
Conclusion
Data minimisation, next to being a legal requirement, offers multiple benefits. It helps to minimise costs, minimise your organisation’s carbon footprint and reduces the risks for your customers. Data minimisation can be applied from the moment a tracking plan is conceived by simply capturing what is required and useful and ensuring that aggregation is used whenever possible. Alternatively a data minimisation audit of your current implementation can be conducted in order to achieve the same result.
Interested? Feel free to reach out !
コメント